Predictive modeling is commonly used to evaluate student performance and identify risk factors at many colleges and universities. Institutions can no longer rely solely on intuition and experience to address the factors influencing student outcomes. A study of Civitas Learning partners found that roughly 40% of students who left college had a GPA of 3.0 or higher—data that typically wouldn’t flag them as at-risk.
While predictive modeling provides early insight into which students might benefit from proactive engagement or supportive resources, concerns are growing that these models may unintentionally reinforce social disparities by predicting worse outcomes for specific racial and ethnic groups.
A recent AERA study, highlighted in the Chronicle of Higher Education, found that predictive models tend to wrongly forecast failure for Black and Hispanic students who actually succeed while overestimating success for White and Asian students. It’s crucial for institutional leaders to really understand the data driving these models to avoid unintentionally reinforcing bias and to ask important questions about how these models are developed and used. Without transparency and a comprehensive approach, predictive modeling can risk making disparities worse instead of helping to fix them.
A Commitment to Fair and Impactful Modeling
Recognizing the potential bias associated with using predictive analytics, like misuse, misinterpretation, and bias, Civitas Learning prioritizes building high-quality models and transparent algorithms. For over a decade, Civitas Learning has been a leader in predictive analytics, developing systems that enhance student success throughout the entire academic journey. Our approach emphasizes that predictive models should be driven by real-time behavioral data, not outdated or static information, ensuring that institutions can make more informed and equitable decisions.
When evaluating your predictive models, consider these three key questions:
1. Do the variables within the model rely on institution-specific data?
Unlike static, demographic-based models, Civitas Learning incorporates real-time behavioral data such as classroom engagement, enrollment patterns, academic performance, and degree alignment. This allows support teams to offer guidance based on behaviors—factors that students can actually change. By combining this with more traditional data like financial aid information, U.S. Census insights, and high school outcomes, institutions can access the most relevant and timely data to drive student outcomes effectively. This equips teams to provide a holistic approach to student support based on timely data-informed actions.
To truly understand what drives success for your unique student population, institutions must identify who is at risk and what factors influence their likelihood of completing a degree. What works for your students may differ entirely from what works at another institution. Tracking key behaviors—like LMS activity compared to peers or card swipe patterns that show resource usage—provides teams with concrete insights to guide proactive support and interventions. However, without transparency and a comprehensive approach, predictive modeling can risk worsening disparities rather than addressing them.
Since students’ needs and performance fluctuate throughout the year, institutions require a model that can adapt and retrain as needed. The model must be dynamic, able to focus on the most relevant data for the institution, and adjust as the institution and its students evolve. Civitas Learning continuously retrains its models, with updates triggered by changes in data mapping or proactive updates from more significant events like changes in degree preferences due to economic shifts.
2. Can you analyze student data granularly to identify trends within specific student groups?
Student outcomes only reveal part of the picture, leaving unanswered questions about which student groups are most affected—both positively and negatively—and why. Institution-specific predictive models can dig deeper and analyze trends and initiatives at a granular level to help institutions provide more targeted and impactful support.
While predictive models aren’t perfect, combining both static and dynamic data—and understanding how they intersect—allows for earlier and more informed interventions. This approach reduces the risk of unintentionally reinforcing bias and increases the likelihood of positively impacting a student’s academic journey.
For example, UTSA uncovered that tutoring and supplemental instruction programs were especially impactful for male and black students. With this insight, the Student Academic Support team launched proactive, targeted outreach for these groups, leading to a 15-point jump in the six-year graduation rate for Black students between 2012 and 2022.
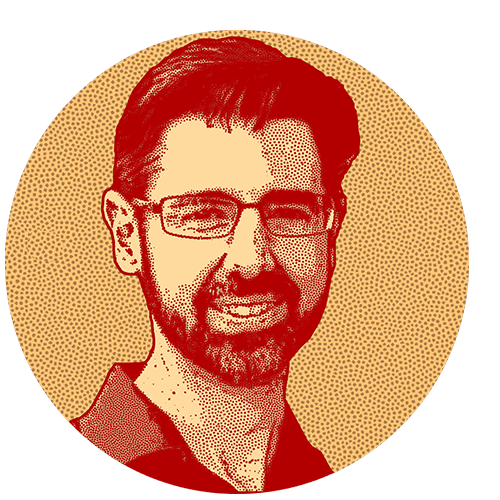
“So much value has come from looking at the different populations of students. What works for one group just does not apply for others.”
—Dr. Mark Appleford,
Vice Provost for Undergraduate Studies, University of Texas at San Antonio
Many institutions use modeling to identify policies that may disadvantage specific student groups. The ability to easily disaggregate data by student group and account for intersectionality between groups has helped Tacoma Community College (TCC) support students more equitably. For example, with their institution-specific predictive models, they can study the effect of drop policies on students by age or ethnic group.
3. How do you combine predictive insights with staff expertise to shape student support efforts?
Predictions are meant to guide timely interventions, but they are projections of what might happen without intervention, not certainties. Simply identifying an opportunity for action isn’t enough. Student Success professionals should use these insights as a starting point for meaningful, compassion-driven student interactions that uncover the root causes of academic challenges.
By treating predictions as signals for support rather than definitive outcomes and understanding the factors influencing them, teams can more effectively connect students with the right resources and services to help them thrive.
For example, relying only on demographic data and national historical trends to guide interventions lacks the precision to pinpoint who to support and when. Institutions need real-time insights into what’s happening during the term to intervene proactively and guide students in the right direction. By combining expertise and professional experience with institution-specific data, institutions are better equipped to take meaningful action, offer more personalized support, and reduce the risk of bias.
Final Thoughts
As AI-powered predictive modeling becomes a central tool in student success efforts, the potential for unintended bias demands careful attention. While these models can offer valuable insights, their true impact lies in how they are built, used, and continuously refined so they can use student data to support students ethically.
Learn more about Civitas Learning’s approach in our whitepaper: Addressing Questions About Bias in Predictive Modeling.