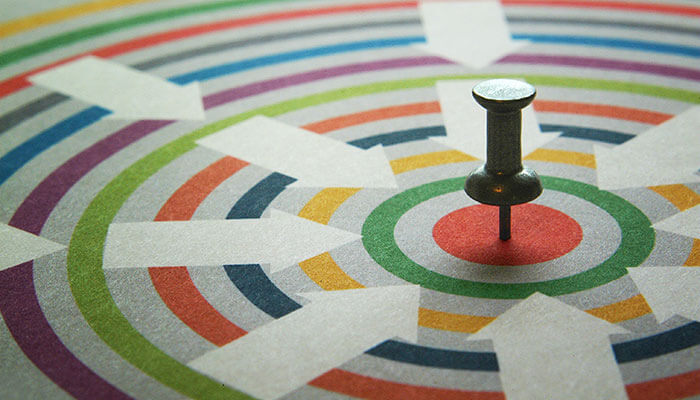
Beyond Intuition: Using Predictive Analytics to Support Students with Precision
Share this Post
When new students arrive each year, it poses new challenges for those who serve and support them. In their book Generation On a Tightrope, authors Art Levine and Diane Dean studied the generation of college students enrolled between 2005 and 2014 to better understand how they were coping with rapid and profound changes. Levine admits that his own theory about these students was flawed, as it was based on the assumption that 9/11 was a defining moment for those students.
Similarly, many assumptions are being made about the impact of the pandemic on students today. The fact that a theorist like Levine can make incorrect assumptions about students raises a question – what assumptions might we be making about the students we serve that could be problematic?
Moving Beyond Intuition
Many who work with students have developed a “sixth sense” for identifying which students may be struggling and what kind of support they need. For instance, student advisors often rely on their intuition and past experiences to determine the risk level a student may face. This approach can be valuable as advisors are familiar with their students and have a deeper understanding of what factors may affect them.
It is important to note that not all students exhibit typical signs of risk or openly present such characteristics. For instance, a study of Civitas Learning partners revealed that approximately 40% of students who left college had a GPA of 3.0 or higher, which is not typically associated with risk identification on campus. Therefore, while educator experience and intuition are valuable, they cannot be the only means institutions use to identify and engage with students.
4 Guiding Principles for Using Student Success Analytics
Considering a Broader Range of Student Data Points
Sometimes, institutions rely on historical data points to analyze previous student trends. However, looking at these static data points alone doesn’t provide a complete picture of the situation. It doesn’t consider the unique behaviors or circumstances of each student, making it difficult to predict their chances of graduating or remaining enrolled. A more holistic approach is required to accurately determine the likelihood of a student persisting or graduating.
One of our partners used to rely solely on academic indicators to determine which students were at risk. However, after implementing our institution-specific model, they discovered that this approach only identified 14% of students who were at risk of not persisting, leaving many others undetected. By incorporating a combination of engagement, behavioral, and academic data, we created derived variables that improved prediction to 83%. This valuable information allows student success staff to act early and support students throughout the semester, helping them stay on a successful path.
Addressing Questions About Bias in Predictive Modeling
Serving Students with Precision
Early and accurate predictions are crucial for ensuring student success. Our partners leverage student-level predictions to provide targeted interventions that maximize their resources and budget. As the data informing risk can change throughout the term, the most successful Civitas Learning partners are the ones who act quickly and implement multi-pronged strategies that enable their teams to scale proactive engagement to the students who need it the most, precisely when they need it.
Intervention campaigns don’t have to be complicated or burdensome to achieve success. Recently, one of our partners planned an outreach program for a group of 300 students who had received a mid-term grade of C- or lower. Rather than sending out a generic communication that might feel relevant to some but not all students, they quickly segmented the students using prediction scores and additional filters (characteristics) to tailor their campaigns with specific, personalized advice. As a result, there was a nine percentage point increase in persistence for those students rated as most at risk of not persisting.
How AI-Based Predictive Modeling Enhances Student Support
Using Analytics to Challenge Our Assumptions
Just like Art Levine, we too can sometimes make assumptions about what students need that may not be accurate. However, our experience working with partner institutions has taught us the importance of using actionable student success analytics to either verify or challenge those assumptions and ensure that we have a data-informed approach to identifying student needs. This is crucial because timely interventions can often be the difference between a student completing college successfully or stopping out. By having access to accurate, real-time signals such as persistence and completion predictions, our team is better equipped to provide focused interventions and support when needed.
Nothing replaces the human intelligence and expertise that you deliver to your students. With actionable student success analytics, your team can easily identify those most at risk and understand why those risks exist- allowing them to intervene in ways that proactively impact student success.